From Lab to Street: How to Unleash the Power of AI on Public Transit
Written by:
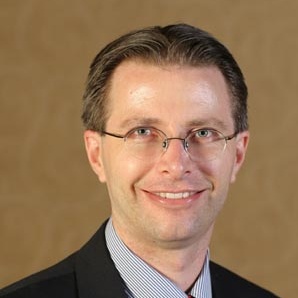
Tom Brodsky
VP, Advanced Technology Group
Written by:
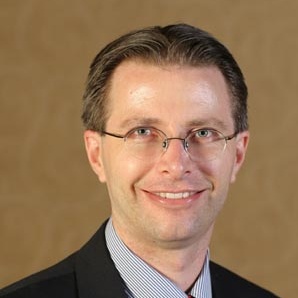
Tom Brodsky
VP, Advanced Technology Group
Over the past decade, improvements in computational power, including Graphical Processing Units (GPUs), dedicated accelerator chips, and new algorithms and learning techniques, have enabled rapid advances in AI and machine learning. This means many real-world problems, including those faced by transit can now be solved by AI running in real-time, even on embedded devices.
Cities are becoming more congested, causing longer transit ride times. Many North American cities average transit bus speeds below 10 miles per hour. Increased congestion and longer ride times are key contributors to the decrease in ridership affecting many North American transit authorities. Congestion also contributes to environmental damage, reduced productivity, lower standards of living, and wasted time and energy.
These real-world problems regularly challenge transit agencies. Taking individual drivers off the streets and helping to make transit more attractive would help solve part of the issue. Helping to make transit routes more efficient would be another.
What if we used technology and AI to focus on the specific challenge of helping to keep dedicated bus lanes clear to meet both of these issues in one approach?
That’s exactly what we did. Today, we will take you on a journey behind the scenes and give you a peek into the crafting, training, and deployment of powerful AI models in the real world for the transit market.
Nailing Down the Vehicle Position: Which Lane Are We In?
Consider the problem of accurately determining the position of a vehicle––which lane is it traveling in? Getting this level of precision is a game-changer, enabling a range of applications from automatic parking violation enforcement to providing invaluable city insights, such as identifying potholes, graffiti, and overgrown trees.
A GPS receiver mounted in the transit vehicle (such as a bus) can provide the approximate position of other vehicles on the road. But it is not accurate enough to determine the driving lane, especially in city centers with many tall buildings. By using the captured footage from a camera mounted in the transit vehicle, an AI model can be created to detect curb locations and the position of the curb. The footage also helps provide additional information to determine the driving lane.
Power Players: Neural Networks and Deep Learning
The most powerful AI models use neural networks and deep learning. A neural network is a computer system designed to simulate the way humans learn and process information. Deep learning is a way computers learn to understand and make sense of really complicated information. Deep learning is not unlike, how we learn from lots of examples to recognize things or make decisions.
By using a lot of examples and GPUs (really fast computer chips), we teach the computer by reviewing the examples many times. It figures out the best ways to make accurate decisions not just for the examples it sees, but also for new situations in the real world.
Think of it like teaching a musician to play a guitar. The musician practices with a vast collection of songs. Through this exercise, the musician learns not only to play those specific songs but also to adapt and play new ones. Similarly, AI models, using deep learning and large datasets, learn to give accurate answers beyond what they were initially trained on.
Diving into the Training Data: The Heart of Model Development
Below is a sample of some images used to train the model for curb detection. In each picture, a real person carefully marks where the curb is in bright green. This is the data the machine learns from. It’s like teaching it to recognize curbs by showing it every possible scenario – empty roads, busy streets with cars parked along the curbs, daytime and nighttime conditions, and more.
Having a variety of scenarios is important. This way, the machine learns to understand curbs so well, it can make decisions on its own regardless of the conditions of the road.
Thousands of Images, Countless Iterations: Model Refinement
When we’re teaching the computer, we give it a whole bunch of pictures, sometimes even tens or hundreds of thousands!
Scientists and developers carefully design the computer’s learning process, sort of like planning a special workout routine. Then, they start the training process, which is like a series of workouts, to make sure the computer gets really good at recognizing objects in the pictures.
This whole process is quite detailed, we’ve simplified it for the purposes of this post.
Putting AI to the Test: How It Performs in the Real World
Once the AI model concludes its training, what comes next? While the team may be happy with the training data results, the proof lies in real-world performance. Testing and validation under realistic conditions is the necessary next step.
The images below show alerts triggered by a system engineered to identify pedestrians in close proximity to transit buses. The system provides early warnings to avert accidents, particularly during left turns.
The outcomes depicted above are commendable, flagging potentially critical situations. Yet, during the early stages of AI model testing, a few scenarios like the ones below also activated alarms:
The Continuous Loop of Improvement
These instances present a challenge! Traffic cones, road markings, poles, and shadows were erroneously identified as people. In these cases, the AI model’s training process is enhanced, or the model’s internal structure is adjusted to boost its capabilities.
These false positives serve as valuable cues—these specific images, along with others resembling them, are incorporated into the training dataset as negative examples. Essentially, the model is explicitly trained to recognize that there are no people in these images, resulting in an enhanced, refined model.
This continuous refinement loop propels the technology to greater power and precision over time.
A Bright Future with AI-Powered Fleet Safety Solutions
In this era of unprecedented technological strides, the potential of AI is boundless. With the fusion of computational prowess, cutting-edge algorithms, and relentless innovation, AI now stands as a powerful force for real-world problem-solving.
Ready to witness firsthand how this groundbreaking technology is ensuring a future of smoother, smarter journeys for all? Learn more about AI-powered fleet safety solutions: https://www.safefleet.net/category/blog/ai/